Combining Machine Learning with IIoT Should Be Your Priority
Machine learning and IIoT are no longer nice things to have, but should be applied together to reap the rewards of cost savings, improved uptime, and performance. In this first of a two-part series on machine learning, Richard Irwin, senior product marketer with Bentley Systems, explains what benefits machine learning can bring to asset-intensive industries, in relation to the Industrial Internet of Things.
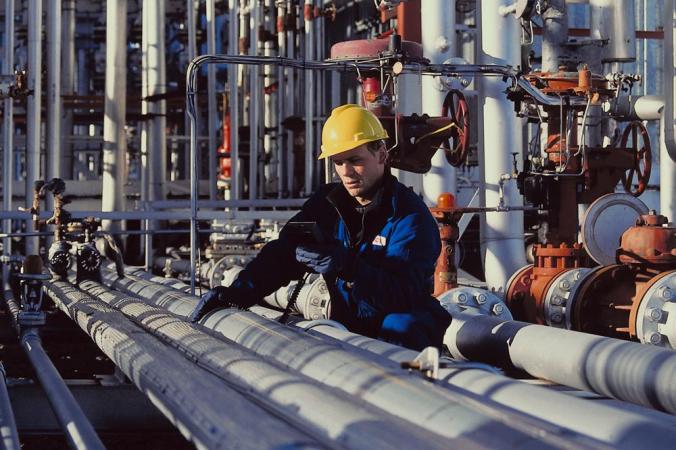
The industrial world is awash with data and new information from sensors, applications, equipment, and people. But the data is worthless if it is left untouched or not used to its full potential with the latest technology. To make the most of big data, industry leaders should implement machine learning alongside the Industrial Internet of Things (IIoT) to take advantage of the benefits increased information can bring to any organization that is asset and data-rich.
We have all experienced some form of machine learning, from streaming movie services that recommend titles to watch based on viewing habits, to banks that monitor spending patterns to detect fraudulent activity. Now, the industrial arena is moving quickly toward using machine learning to take advantage of the Industrial Internet of Things.
As the velocity and variety of data becomes available through advancements in sensor technology to monitor just about anything, machine learning is being applied to efficiently manage increasingly large and fast-moving data sets. Machine learning can handle large and complex information to discover otherwise hard-to-see patterns or trends in the data, such as normal behaviour and anomalies. It can then learn these patterns and apply it to new data to detect similar patterns in the future. An example would be to model the performance of a piece of equipment, such as a pipe, in relation to the temperature of its surroundings. Machine learning can be taught to see what normal behaviour looks like, and by applying the model to current data, it can spot abnormalities, such as when the pressure within the pipe increases while the temperature remains the same. The system can then predict from existing knowledge that something is not right and send out notifications.
Demystifying Machine Learning
A subset of artificial intelligence, machine learning uses algorithms and models to derive insights from multiple sources, including sensors, mobile devices, and computer networks. While traditional analytical methods like business intelligence and predictive analytics have paved the way for deriving insight from data, machine learning takes it further by interpreting the data rather than interpreting human participation. Predictive analytics can answer questions such as, “If I increase production by x percent, how will it affect the bottom line in the next quarter?” Whereas machine learning focuses on the outcome and teaches a computer to automatically uncover the multiple and complex factors that lead up to it. This points to a far more accurate predictive model that can automatically adjust over time through learning. The more data that is analyzed, the more accurate the predictive model.
To Stay Competitive, We Need Machine Learning
Unlike business intelligence and predictive analytics methods that require a significant amount of manual labour and time, machine learning automatically produces insights at a consistent and accurate rate. Machine learning detects data that is out of sync, like a piece of equipment that is underperforming and could potentially fail. It is then able to add this piece of information and learn from it in future models. This makes decision-making easier and more reliable.
In any industry, the ability to recognize equipment failure, and avoid unplanned downtime, repair costs, and potential environmental damage, is critical to success. This is even more relevant in today’s turbulent times. With machine learning, there are numerous opportunities to improve the situation. Three of the main forms of predictive analysis are prediction maintenance (failure prediction), forecasting (demand), and workforce management.
Predictive Maintenance – One of the most applicable areas where machine learning can be applied within the industrial sector is predictive maintenance. Predictive maintenance is the failure inspection strategy that uses data and models to predict when an asset, or piece of equipment will fail so that mainten ance can be planned. Predictive maintenance can cover a large area of topics, from failure prediction and failure diagnosis, to recommending mitigation or maintenance actions after failure. The best maintenance is advanced forms of proactive condition-based maintenance. With the combination of machine learning and maintenance applications leveraging IIoT data, the range of positive outcomes and reductions in costs, downtime, and risk are worth the investment.
Demand Forecasting – Accurately forecasting high levels of demand, such as within a utility service, gives a company a competitive advantage. It provides them with the information they need to meet customer demand by anticipating future demand or consumption. In the energy sector, for example, storing energy is not cost-effective, so power companies need to forecast for future power consumption, so that they can efficiently balance the supply with the demand. This sector is faced with twin problems associated with outages in peak demand, while too much supply leads to wasted resources. With advanced demand forecasting techniques, utilities can ascertain hourly demand and peak hours for a day, allowing them to optimize the power generation process. Using information such as historical demand data, regions, population, weather patterns, events, and so on, organizations can predict demand on any day or period in the future. This is essential for ensuring the utility can produce the resource in time.
Workforce Management – In workforce management, the aim is to optimize the workforce and make it as productive as possible while reducing labour costs, travel, additional call outs and improving customer service. There are measures in place to help with smarter scheduling (mapping the right person with the right skills to the right location with the right tools and the right permits) and route optimization to reduce travel and costs. These are still done manually in some cases, and are error-prone. Machine learning can do this automatically, and by incorporating historical data of previous jobs, weather, and time of year, it could gauge where problems might arise.
Summary
With the arrival of the Industrial Internet of Things, data is growing and becoming more accessible. With the ability to acquire more data, more advanced technologies are required to scrutinize and filter out the important information and value held within. But, it can only be exploited by identifying what works well and what does not. Machine learning features complex algorithms to sort through large amounts of data, identify patterns and trends within it, and make predictions. Key decision makers need to take advantage of this “match made in heaven” to optimally control and manage their assets – get on top of it now before asset data is unmanageable. Preventing assets from failing, forecasting demand, and optimizing movements of your workforce, are just a few of the machine learning techniques that can be applied to improve the overall performance of the organization. By combining these elements and more, machine learning will bring, as it matures, significant benefits. The “Industrial Internet of Things” and machine learning should no longer be considered just buzzwords – instead, put together, they should be your number one priority.
Five questions to ask before investing in machine learning:
- Question your data – What do you need to know, what are you looking for exactly? What do you want your data to tell you? What aren’t you seeing what you hope the data can provide?
- Is your data clean? – Make sure your data is available, ready and validated; the more data the better and the more accurate the outcomes will be.
- Which ML platform do I choose? – Choose your machine learning platform carefully considering interoperability.
- Do I hire a data scientist, and how do they integrate? – With machine learning, there might be a need for a data scientist or analyst, but they shouldn’t be locked in a dark room.
- Can I share the data output? – Knowledge gained through machine learning should not just be applied to one project at a time. Its scalability means it can and should be incorporated across the whole enterprise, delivering insight into any area rich in data. Plan to get the most out of machine learning.