Black Swans in Maintenance and Industrial AI: Predicting the Unpredictable?
Maintenance disasters caused by unexpected events, generally called “black swan” events, may be prevented by the development of Industrial AI (IAI), given its ability to find “invisible” insight in data. For centuries, swans were considered to be white, but in 1967, a black swan (Cygnus Atratus) was discovered in Western Australia.
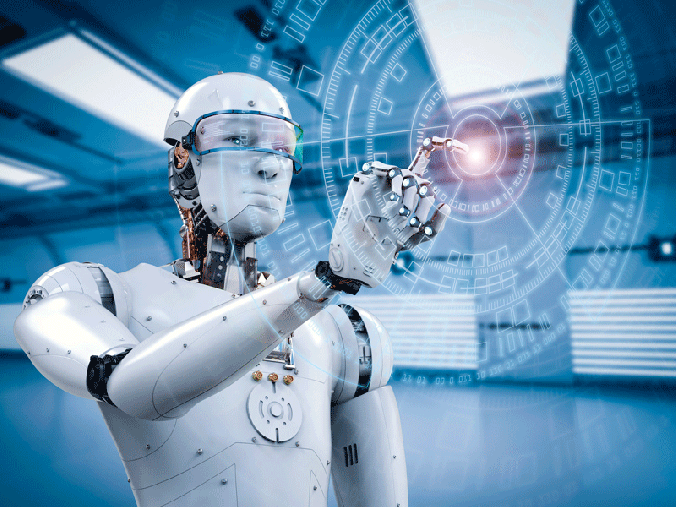
The term “black swan” became a metaphor for a supposed impossibility that was contradicted by new information. Black swans are recognized in diverse fields, including finance, history, science, and also technology. Their common attributes across fields are the following: a) they have extreme impacts; b) they lie outside the realm of regular expectations; c) they are unpredictable (with the knowledge constraints of each domain) and d) they appear stochastically.
The operation and maintenance community also encounters black swans. Generally speaking, they deal with the impacts of extreme natural degradation and man-made accidental or malevolent intentional hazards for critical facilities by taking a risk-based approach, where risk is a function of the likelihood of event occurrence and the resulting consequences. However, black swans are not foreseeable by the usual calculations of correlation, regression, standard deviation, or reliability estimation, and prediction. In addition, expert opinion has minimal use, as experience is inevitably tainted by bias. The inability to estimate the likelihood of a black swan precludes the effective application of asset management and risk calculation, making the development of strategies to manage their consequences extremely important. In the coming years, Industrial AI empowered digitalization may be able to cope with the potential or actual consequences of these unforeseen, large-impact, and hard-to-predict events.
Vulnerability of Assets to Black Swans
Complex systems that have artificially-suppressed vulnerability tend to become extremely fragile, while at the same time exhibiting no visible risks. Although the intention of maintenance stakeholders is to keep these assets available, reliable and non-vulnerable, the result can be the opposite. These artificially-constrained systems may become prone to unpredictable black swans. Indeed, observing normality, maintenance engineers tend to believe that everything is fine. However, environments with “artificial normality” eventually experience massive blow-ups, catching everyone by surprise, and undoing years of failure-free maintenance.
The longer it takes for the blow-up to occur, the greater the resulting harm. If anything had indicated the need for protection, maintainers would obviously have taken preventive or protective actions, stopping the black swan or limiting its impact.
It is unfortunate that we cannot develop convincing methods to infer the likelihood of a black swan from statistical-inductive methods (those based on the observation of the past) and combing this with statistical deductive methods (based on known valid laws and principles) to derive the likelihood of a future event based on the findings. This is especially problematic in maintenance. Arguably, Industrial AI has the potential to change all this.
Industry 4.0 and Black Swans
In the technology industry, every new mobile App, computer program, algorithm, machine learning construct, etc. is advertised as revolutionary and destined to change the world. However, black swans still exist and are highly impactful, especially in a connected world. Industry 4.0 technologies must learn to handle them.
The knowledge cycle refers to the frameworks or models used by organizations to develop and implement strategies, including in maintenance. The knowledge cycle or the knowledge management cycle (or knowledge life cycle) is "a process of transforming information into knowledge within an organization which explains how knowledge is captured, processed, and distributed in an organization." Today’s organizations must deal with increasingly complex problems. The rapid changes in the economy and a highly competitive market lead to uncertainty, making it important to predict possible outcomes or events to remain operational. In addition, there is a need to develop knowledge life cycle strategies to recognize the possibility of an unlikely critical situation – this, of course, is not easy, but organizations have access to immense knowledge. This knowledge should be managed systematically to identify and eliminate unpredictable events or reduce the consequences.
The Industrial AI learning framework aimed to turn black swans in maintenance to white swans is shown in Figure 1. As the figure shows, it is a mixture of various conventional knowledge cycle models. This integrated knowledge cycle model suggests a way to find black swan events, create a strategy to prevent them, and to incorporate that strategy. The framework covers two major areas of knowledge: known and unknown. The conventional cycle steps of known knowledge include knowledge capture and creation, knowledge dissemination, knowledge acquisition and application, knowledge base updating. Black swan events are an example of unknown knowledge. The cycles of known and unknown knowledge move at the same pace and merge at the end with the main goal of recognizing a black swan and resisting it. The resulting white swan or new known knowledge allows the exploration of previously unknown areas.
Black Swan and Anomaly Detection
In statistical terms, a black swan corresponds to the disproportionate contribution of a few observations to the overall picture. In maintenance, a few observations can constitute the normality, the information provided by outliers may be missed, and the resulting reduced data set of failure modes will neglect the total. Even a simple underestimation of the required sample size can cause a black swan.
Maintenance engineers use stochastic processes and such tools as reliability estimation to predict the behaviour of assets, but the excessive application of the “law of large numbers” is not advisable. Simply stated, the law of large numbers indicates that the properties of a sample will converge to a well-known shape after a large number of observations. Although bigger datasets of faults lead to greater accuracy and less uncertainty when predictive maintenance is performed, the speed of convergence (or lack of it) is not known from the outset.
Outliers are considered by modelers in risk management, but they cannot capture off-model risks. Unfortunately, in maintenance engineering and asset management, the largest losses incurred or narrowly avoided by maintainers are completely outside traditional risk management models.
Predictability of Black Swans
Given the subjectivity of human decision-making, incorporating the use of AI modelling as a tool could positively impact outcomes and support maintenance expertise. Arguably, using data-driven approaches increases objectivity, equity, and fairness. Machine learning can quickly compile historical data and create a risk map to assist with decisions. In addition, using a predictive model that has a learning component can account for variations in different subpopulations and potentially capture changes in risk over time.
Artificial intelligence has the potential to positively influence maintenance effectiveness; however, when used inappropriately, there is a risk of AI technology underperforming due to lack of knowledge. There is a fine line between bias and prediction, when using past information to make decisions on future behaviours. It may be impossible to account for all unknown factors that could influence the model, particularly when future events do not follow the historical data, rendering the model invalid; prognosis based on sensor data and past knowledge might therefore be useless, and predictions will be affected by long tails (black swans), as shown in the figure below.
Unanticipated events with a major impact could weaken the predictability of the model. Dataveillance refers to the systematic monitoring of assets using data systems to regulate asset behaviour in maintenance field. It is another concern when using a predictive model. In particular, using the model to monitor or surveil something is highly contested. Therefore, it is imperative to understand and account for potential biases when using a predictive model. For example, biases in favour of positive results could impact the interpretation of the data, i.e., looking for data to justify decisions instead of justifying decisions based on the data.
AI algorithms are not generally biased, but the deterministic functionality of the AI model is subjected to the tendencies of the data; therefore, the corresponding algorithm may unintentionally perpetuate biases if the data are biased. Biases in AI can surface in various ways. For example, the data may be insufficiently diverse, prompting the software to guess based on what it “knows.”
There are four basic types of bias associated with AI. First, interaction bias occurs when the user biases the algorithm through interactions. For example, the user may provide vibration data but not data on other failure modes; when a misalignment appears, the algorithm may not recognize the failure. Second, latent bias occurs when the algorithm incorrectly correlates parameters and condition indicators. Third, selection bias occurs when the data used to train the algorithm over-represents one population, making the algorithm operate better for that population than for other populations. This is typical of dominant failure modes which may hide the non-dominant modes, even though the latter eventually may have a higher impact. Fourth, lack of relevant data due to changes in the asset configuration, which makes the learning loop unactualized.
Asset managers need to cultivate a culture of resilience, i.e., the capacity to absorb disturbance while retaining a basic function and structure. Traditional reliability and maintenance decisions are often tainted by personal biases and based on a limited time span of observations. Engineers do not like uncertainty and ambiguity, so they focus on specifics instead of generalities and look for explicit explanations. Such thinking is shaped by their training in linear logic. But nearly all black swan events involve complex causal relationships.
Industrial AI must compensate for the human blindness to black swans. First, humans tend to categorize, focusing on preselected data that reaffirm beliefs and ignore contradictions. Second, humans construct stories to explain events and see patterns in data when none exist, due to illusion of understanding. Third, human nature is not programmed to imagine black swans; humans tend to ignore the silent evidence and focus disproportionately on either failures or successes. Finally, humans overestimate their knowledge and focus too narrowly on their field of expertise, ignoring other sources of uncertainty and mistaking models for reality.
As black swans are not predictable, and humans are both limited and biased; Industrial AI must propose a way to manage black swans by determining the emerging patterns and disrupting undesirable patterns while stabilizing desirable ones. A host of studies demonstrate the human tendency to assign patterns to random data and create descriptive narratives, resulting in a focus on the mundane and missing the extraordinary. Pitfalls of making projections from limited data include the failure of many assets.
Maintenance engineers are not well-equipped to deal with anticipation, waiting for an important event that will occur infrequently, if at all. In a few extremely conservative sectors, like nuclear energy, maintainers are tasked with taking action during incidents that may never happen. Numerous cases can be cited in the airline and marine industries, where nothing out of the ordinary is observed for long periods, but a deadly combination of fatigue and boredom ultimately leads to a catastrophic failure. Engineers also have a tendency for tunnel vision, focusing on the known sources of uncertainty and ignoring the complexity of reality. As events that have not taken place cannot be accounted for, they do not have adequate information for prediction, particularly since small variation in a variable can have a drastic impact. It is not the random uncertainty of probabilistic models, often called “known unknowns” or “grey swans” but the uncertainty due to lack of knowledge, i.e., unknowns or black swans, that is the main concern. No probabilistic model based on in-box thinking can deal with out-of-box events.
Uday Kumar, Diego Galar, and Ramin Karim